Deploy AI To Become A cGMP "Special Agent" With A License To Care
By Ajaz S. Hussain, Ph.D., independent subject matter expert
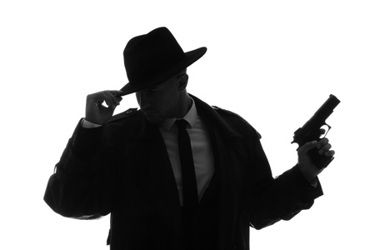
The pharmaceutical industry struggles with current good manufacturing practices (cGMP) violations and other systemic issues. cGMPs are foundational standards ensuring drug quality and safety. Deficiencies in quality systems, inadequate training, and recurring cGMP failures compromise public health, disrupt supply chains, and undermine industry credibility. Adding to these challenges are incomplete product recalls and concerns over the effectiveness of regulatory oversight.
Addressing these systemic issues requires more than technical fixes — it demands cultural and organizational transformation. While not a panacea, artificial intelligence (AI) presents a powerful dual-edged tool. Thoughtfully applied, AI can empower professionals to become "special agents" of quality, equipped with a "license to care." This transformative approach, I posit, can enhance compliance and foster a culture of accountability, continuous learning, and innovation.
At The Heart Of cGMP Compliance: The Human Factor
At the heart of cGMP compliance lies the human factor. Regulations such as 21 CFR 211.25 emphasize the need for education, training, and experience.1 Yet, in practice, reliance on formal qualifications often falls short. Inconsistent experiential learning and outdated or invalid procedures exacerbate quality issues. Ignorance and fear-driven behaviors can lead to breaches in [the assurance of] data integrity (BAD-I),2 often overlooked by immature quality management systems. These failures typically surface during inspections, resulting in FDA Form 483 observations or warning letters highlighting lapses too late to prevent harm. The industry's reliance on reactive measures perpetuates inefficiencies, turning regulatory compliance into a "cost of doing business" rather than a commitment to excellence.
Empowering Professionals Through Self-Authorship
Fostering “self-authorship”3 in every job responsibility is a responsible solution to systemic cGMP challenges. With this mindset, professionals critically assess and refine processes while owning their roles in assuring quality over a product's lifecycle. This stage of professional development aligns with frameworks like "Breaking the 2-3 Sigma Barrier," promoting operational maturity and continuous improvement.4 Self-authorship entails “interval validation” and minimizes dependence on external audits, empowering professionals to address systemic inefficiencies proactively.
However, achieving this transformation is particularly challenging in the global pharmaceutical supply chain, where diverse cultural, linguistic, and economic contexts intersect. The temptation to ignore issues — “If I don’t look, there is no problem” — prevails, perpetuating quality gaps. Encouraging critical thinking and accountability at every level is essential for overcoming these challenges.
AI: A Catalyst For Transformation
When thoughtfully implemented, artificial intelligence can become a transformative tool for promoting self-authorship by integrating cross-functional information, fostering design thinking, and advancing a systems approach to quality management.5 Traditionally, siloed information and the division of expertise and labor have made it challenging to address interconnected issues holistically. Without such integration, teams often fail to discern common causes of problems, focusing instead on superficial special causes.6
Implementing AI thoughtfully involves designing its utility in the quality management system with a thorough understanding of the specific pharmaceutical practices to be improved. Validating these practices and continuously monitoring their performance is essential. This approach ensures that AI outputs are reliable, interpretable, and compliant with regulatory standards. Through such implementation, organizations are empowered to self-author improved practices in root cause investigations, democratize decision-making closer to front-line executive levels, and move beyond the "root cause unknown" mindset — avoiding undue blame on analysts or operators, and fostering systemic accountability.
Misuse or overreliance on AI systems can lead to unintended consequences, such as erroneous decision-making due to incorrect training data. Additionally, poorly designed or implemented AI systems can create a false sense of security, where professionals may trust output without adequate validation or oversight. To mitigate these risks, organizations must ensure transparency in AI processes, foster a culture of critical evaluation, and maintain human expertise as an integral part of quality management. Adequate safeguards, regular audits, and rigorous testing are essential to realize AI's benefits while minimizing risks.
Using AI To Mitigate Dissolution Failures
For instance, recurring dissolution failures can stem from various sources, including poorly defined specifications, inadequately developed testing methods, actual product failures, and errors of commission. In commercial operations, the scope of root cause investigations typically focuses on “errors of commission,” doing something that one should not have, i.e., a deviation. At the same time, the validity of the dissolution method and the appropriateness of regulatory specifications or product and process design are rarely questioned.7 Such failures are ingrained in the mindset that it is “FDA approved” and “validated,” so “if I don’t look, there is no problem.”4 AI can overcome this mindset and promote a product life cycle approach, analyze variability in testing conditions, recognize inconsistencies, and predict outcomes, enabling more effective quality by design (QbD) practices.5
While at the U.S. FDA, I witnessed recurring dissolution failures, including cases with national security implications. In one notable instance, a legacy product's incidental eruption of out-of-specification dissolution test data prompted severe regulatory enforcement action that threatened the product's supply. The only negotiable solution was to let production continue with a commitment to reject/recall failing batches. At the same time, the company committed to completely redesigning the product and manufacturing process — a lengthy and resource-intensive undertaking.7
Could AI have mitigated this situation? While I was at the FDA, large language model (LLM)-based AI didn’t exist. Although we had attempted to apply machine learning, these efforts were not scalable.7
In a recent experience using a LLM trained on FDA guidance, key scientific literature, and my previous presentations, the LLM demonstrated an impressive ability to process information, identify patterns, and propose actionable insights that often surpass those of many professionals I have encountered.5 Its effectiveness could be evaluated when employed in a critical thinking mode by asking relevant questions, assessing the patterns the AI has recognized, and identifying instances where it may be hallucinating. In this way, AI can integrate cross-functional information, foster design thinking, advance a systems approach to quality management, and address interconnected issues holistically. While human expertise is irreplaceable in providing context and intuition, AI complements these strengths by streamlining data analysis, highlighting inconsistencies, and accelerating problem resolution.
Using AI To Avoid Adverse Outcomes With Facility Inspections
W. Edwards Deming famously advised, "Cease dependence on inspection to achieve quality."8 Inspections, while critical, are reactive and often too late to prevent systemic failures. Observational findings from FDA inspections highlight persistent gaps in cGMP compliance. What about in a global supply chain where many facilities remain uninspected?
A recent investigation revealed that contaminated eye drops produced by an uninspected facility in India led to multiple deaths and injuries in the United States. Inspections of the facility uncovered unsanitary conditions and detected positive bacterial test results from environmental samples taken in critical drug production areas.9
In another case, a major generic drugmaker issued several recalls for “pills that didn’t dissolve properly.”10 Moreover, the investigative report highlights that the FDA has not inspected the factory since the recalls. What another FDA inspection might reveal remains concerning — even though the product in question has been FDA-approved and its manufacturing process validated previously.
Adopting a product life cycle approach to investigations, corrective and preventive actions, and overall quality management is essential to addressing common causes of external failures and preventing their recurrence. The challenge we face is that we often fail to distinguish between common and special causes of variation.8 Understanding the differences between these types of variation, their causes, and how to predict process trends is essential for management to address and eliminate problems or barriers within the system effectively.
Recurring cGMP violations and external quality failures emphasize the need for increased oversight and systemic improvements. Focusing on common causes of failures is crucial to achieving systemic enhancements. While some industry segments are moving toward continuous manufacturing with real-time monitoring and control, many areas and legacy products remain susceptible to recurring external failures and eroding assurance of therapeutic equivalence.11 In this context, well-implemented AI can be a valuable and transformative tool. It can promote self-authorship by integrating cross-functional information, encouraging design thinking, and advancing a systems approach to quality management.12
Since the COVID-19 pandemic, approximately 2,000 pharmaceutical plants have not been inspected in five years.13 Increasing geopolitical tensions and strained relationships have also made the global pharmaceutical supply chain vulnerable. Given the prevailing gaps in ensuring the quality of narrow therapeutic index drugs,14 the risk of catastrophic failures is concerning. There is an urgent need for grassroots mitigation strategies to address these issues effectively. Leveraging AI for proactive quality management can play a pivotal role in bridging these gaps.
Fostering A "License To Care"
The journey toward systemic cGMP compliance begins with a cultural shift. Professionals must transcend blind compliance and develop critical thinking and accountability. AI offers the tools to enable this transformation, bridging knowledge gaps and fostering a proactive approach to quality management.
By integrating self-authorship into professional development and leveraging AI to improve processes continuously, the pharmaceutical industry can instill a culture of accountability. This evolution is about more than meeting regulatory requirements—it is about empowering every professional with a "license to care."
To safeguard public trust and health, we must elevate every practitioner's role to that of a special agent in the cGMP environment. By combining the transformative power of AI with self-authorship principles, we can build an industry that prioritizes quality, principled compliance, and care. Let this be our shared mission: to make pharmaceutical excellence a mandate and a commitment to care.
References
- Hussain, A. S. Education, Training, Experience & 21 CFR 211.25: Theory, Practice, and Epistemology | LinkedIn (March 24, 2016).
- Hussain, A. S. Pharmaceutical Hurricane Named BAD-I | LinkedIn (3 September 2017).
- Kegan, R. In Over Our Heads: The Mental Demands of Modern Life. Harvard University Press. Publication date: 07/21/1998
- Hussain, A. S. How To Break The Pharmaceutical 2-3 Sigma Barrier (Like Amgen), Pharmaceutical Online, Guest Column | September 18, 2017.
- Hussain, A. S. Can an AI learn better than most from my past presentations on Dissolution testing? | LinkedIn (December 26, 2024).
- Hussain, A. S. Pharmaceuticals Beyond 2020: Professionals and Artificial Intelligence. Indian Pharmaceutical Association. Pharma Times. 52: 14-19 June 2020. https://ipapharma.org/3d-flipbook/pharma-times-june-2020/
- Hussain, A. S. Past, Present, and Future of Pharmaceutical Dissolution Testing: A Disciplined Reflection and Synthesis. Society for Pharmaceutical Dissolution Science, eDisso Newsletter, 3-7, September 2019. https://spds.in/wp-content/uploads/2020/07/Final-e-DissoNews-Letter-2019-sept.pdf
- Deming, W E. Out of the Crisis. The MIT Press. January 2000.
- Recalled contaminated eye drops linked to bacteria have led to a fourth death, CDC says. USA Today. May 20, 2023.
- The FDA Hasn’t Inspected This Drug Factory After 7 Recalls for the Same Flaw, 1 Potentially Deadly. by Patricia Callahan, Debbie Cenziper, and Megan Rose, ProPublica, December 12, 2024.
- Hussain, A.S., Gurvich, V.J. & Morris, K. Pharmaceutical “New Prior Knowledge”: Twenty-First Century Assurance of Therapeutic Equivalence. AAPS PharmSciTech 20, 140 (2019). https://doi.org/10.1208/s12249-019-1347-6
- Hussain, A. S. Formulating with Artificial and Natural Integrity Fifth Order of Development. VAMs 2.0 AI-Enabled Development and Commercialization Conference, October 2024
- Nearly 2,000 drug plants are overdue for FDA checks after COVID delays, AP finds | The Associated Press
- Physicochemical failure modes for Narrow Therapeutic Index (NTI) drugs. European Pharmaceutical Review. 2 July 2021. By Dr. Ajaz Hussain, Dr. Harsh S. Shah, Dr Kaushalendra Chaturvedi, and Dr. Kenneth Morris
About The Author:
Ajaz S. Hussain, Ph.D., is a pharmaceutical quality and regulatory science expert with decades of experience across academia, industry, and government. As a former deputy director of the FDA's Office of Pharmaceutical Science, he was pivotal in pioneering initiatives like the process analytical technology (PAT) framework and the Pharmaceutical Quality for the 21st Century Initiative. Hussain also served as president of the National Institute for Pharmaceutical Technology and Education (NIPTE), advancing pharmaceutical technology and regulatory science. Now an independent consultant, he empowers organizations to integrate design thinking with a systems approach to advance innovative technologies that hold high potential for enhancing patient care and operational excellence.