Dispelling 4 Common Myths Of Data Quality Governance
By Steve Gens and Preeya Beczek
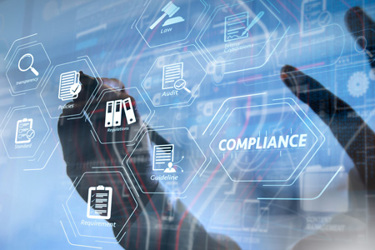
From the latest measures around safety and regulatory rigor to renewed focus on agility, efficiency, and streamlined paths to market, a growing number of routine processes in life sciences rely on companies’ effective organization and handling of data.
It's in this context that the concept and discipline of data quality governance come to the fore. The more critical data becomes to regulatory procedures, to safety processes, to clinical research, to manufacturing, and ultimately connecting all of those parts of the end-to-end value chain more seamlessly, the greater the need for formal strategies and provisions around the governance of that data’s quality across all internal and external touchpoints.
The top performing companies, in Gens & Associates’ 2022 in-depth World-Class RIM survey of 76 life sciences organizations, indicated that they will have most of their systems connected and sharing data within the next two to three years. These include electronic trial master file (eTMF) systems, quality management systems (QMSs), master data management (MDM), and enterprise resource planning (ERP) systems among the highest priorities for investment.
But this places renewed pressure on these companies to increase and assure the quality and dependability of the underlying data — and getting to this point can seem a daunting undertaking.
Achieving robust data quality governance needn’t be an unwieldy prospect, though. In reality, the most common preconceptions don’t stand up:
Myth 1: Complexity & High Cost Are Unavoidable.
Remember that positive change must start somewhere, so decide whether a top-down or a function-by-function (with consistent practices) approach will produce the quickest wins and the greatest overall progress. In a large organization with a vast product portfolio, starting in one department, learning and applying any resulting lessons, and then rolling out more widely might work best, for instance, while in an emerging company it might make more sense to drive improvements broadly from the top.
The data driven agenda might feel fresh and new in life sciences, but digital process transformation is well advanced in other industries and solid frameworks already exist and have been adapted for data quality governance in a life sciences “regulatory+” context. In other words, this needn’t be a steep learning curve or leave companies with huge holes in their transformation/organizational change/IT budgets.
Myth 2: You’re Doing This Largely For Regulatory Compliance.
Regulators from the EMA in Europe to the FDA in the U.S. are making proactive strides toward data standards such as the Identification of Medicinal Products (IDMP) as the currency of new filings and ongoing information exchanges. These requirements might seem to be the most obvious driver for getting your company’s product and manufacturing process-related data in order. Yet there are many higher purposes for making data-related investments. These range from more tightly run business operations to a safer and more convenient experience for patients as consumers of existing and new products.
A commitment to data quality and consistency allows a company to issue and maintain more of its guidance in digital form for customers and end users, for instance, so that they can look this up at any time, wherever they are in the world, knowing that the information is correct and up to date.
Myth 3: This Is An IT/Data Management Concern First And Foremost.
Time and again, the key success factors for a data quality program are found to have little to do with technology and everything to do with culture, organization, and mindset.
Specific contributors to progress, distilled from the most promising programs being rolled out today, include a shared data quality vision — so that good data-related practice becomes second nature among everyone who touches that data (from those working in the labs or running clinical trials to those pulling information together for regulatory purposes); the proactive appointment of a data quality office and officer; a culture of data quality built into rewards systems; and formal continuous improvement.
A lot of what’s needed has to do with nurturing the right culture, assembling the right teams or assigning key roles, transparency of data quality performance (communicating both progress and successes), and being on the same page as a company about the goals of this whole undertaking.
Myth 4: You’re Already Vigilant About Data Quality So You Don’t Need A Formal Program.
Most companies have challenges with data quality, which will deepen as data becomes increasingly fundamental to critical everyday processes.
Here’s a quick checklist that will soon highlight when a company has challenges with its data quality (which will deepen as data becomes increasingly fundamental to critical everyday processes):
- Data quality is not viewed as an organizational competency or linked to the organizational culture;
- There is no clear data quality vision, policy, or strategy;
- There is no overarching data quality operating model;
- There’s a lack of transparency of the current/evolving data quality status and any trending/reporting;
- Data quality is not built into rewards or recognition systems; and
- Data connectivity is being prioritized ahead of the organizational support required to properly manage and leverage the value of connected data.
The 3 Phases Of A Good Data Quality Governance Program
With a strong three-phase framework, any company can start to cover good ground, however you decide to approach this (e.g., function by function, or top down and enterprise wide):
- The Establish/Launch Phase. This initial ground preparation phase is about setting out a data quality vision and principles; establishing an actionable data quality operating model with formal jobs and roles; and conducting an awareness campaign.
- The Operational Phase. This involves establishing optimal processes and capabilities — e.g., by adjusting to learnings from the Establish/Launch phase; ensuring that all roles with a bearing on data quality have these responsibilities set out in job descriptions and covered as part of the annual review process; and establishing recognizable rewards for high-quality data.
- The Optimization/Institutionalization Phase, in which desirable behavior is embedded and fostered within the organizational culture, ensuring that everyone gets and stays on board with maintaining and continuously improving data quality, to everyone’s benefit. Tools might include automated data quality dashboards to monitor KPIs; data integration and connectivity throughout function and organization; and organization-wide data quality level reporting, supporting a culture of quality.
Taking a phased approach to systematic data quality governance paves the way for your company to move forward with your improvement efforts now, taking a bite-sized approach. As progress is witnessed, momentum should gather organically.
About The Authors:
Steve Gens is the managing partner of Gens & Associates, a global life sciences advisory and benchmarking firm specializing in strategic planning, regulatory information management (RIM) program development, industry benchmarking, and organizational performance. He can be reached at sgens@gens-associates.com.
Preeya Beczek, director of Beczek.com, is an independent regulatory affairs expert, providing teams with insights, advice, and strategies for operational excellence by optimizing processes, systems, roles, and operating models. Beczek can be reached on LinkedIn.