How To Close The Looming Life Sciences Data Storage Gap
By Thomas Armel, Ph.D., EY-Parthenon
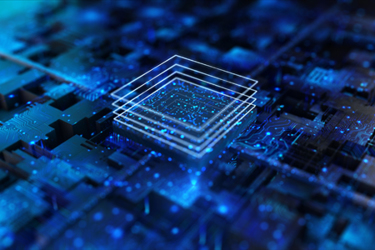
Organizations across life sciences are facing a data storage dilemma: how to balance the need to store and potentially monetize mushrooming amounts of data while managing IT budgets and limiting the total cost of ownership (TCO). By 2030, the global demand for storage will exceed 32 million petabytes, with each individual petabyte being enough capacity to store more than 223,000 DVD-quality movies. At the same time, forecasted shipments of storage media, such as tape and hard disc drives, are not keeping pace. In fact, in the healthcare industry, the amount of data generated already exceeds storage capacity by a factor of 2.5.
These data assets and the products that utilize them, such as artificial intelligence, underlie potential monetization opportunities and influence the cadence of innovation cycles. However, the systems and architectures used to store, manipulate, and report on these data contribute to costs. Streamlining access to data and analytics tools can reduce resource demand and help quicken the time to market.
Not addressing the data storage issue may impede revenue growth, increase expenses, and delay the timeline of bringing new products and services to market. Life sciences companies should begin now to develop strategies to reduce this gap through data prioritization, revamping data storage architectures, and application of emerging technologies. From understanding the size of future data storage needs to considering strategic partnerships, the practical steps we outline are applicable to any life sciences organization looking to harness and store increasing reams of data.
Causes Of The Increasing Data Storage Need
Several factors contribute to the growing gap between what companies need to store and what can be stored economically. Data archive sizes are increasing and being stored for longer time periods, from large scientific projects such as UKBiobank and the Million Veteran Program to medical imaging and longitudinal patient data collection. Additionally, whole-genome sequencing data coupled with AI/ML-powered association studies and biobank cohorts has enabled quantification of the role genetic variation plays and has given rise to the clinical implementation of genomic screening.
Another reason for increasing data storage in life sciences is a widespread hesitancy to delete what at first glance is valueless data. Ever-evolving machine learning algorithms may be able to utilize this data to improve patient outcomes, while future ecosystems may provide as-of-yet undeveloped data monetization opportunities. There is also a growing need to maintain redundant copies to address challenges from error or cyberattack recovery and to mitigate litigation-associated risk.
In some instances, inefficiencies in legacy storage architectures can lead to further accumulation of redundant data. Additionally, government regulation, such as the Healthcare Insurance Portability and Accountability Act (HIPAA) in the U.S. and the Records Management Code of Practice for Health and Social Care 2016 in the U.K., impose requirements for security, compliance, and reporting. These regulations call for data retention periods that can span decades.
Scaling Limits Will Affect Total Cost Of Data Ownership
The final factor that accounts for the gap is the scaling limits of storage media and the implications this has on the capability for storage manufacturers to meet the growing demand. Historically, data was stored within an organization’s data center on-premises in a hierarchy with high-cost, high-performance, low-capacity media at the top (hot tier), followed by two lower tiers (warm and cold) that increase in capacity with lower cost and performance. The hot tier was dominated by designed dynamic random-access memory (DRAM), followed by spinning hard disks in the warm tier and tape in the cold, or archival, tier.
Following Moore’s Law, technology advances over the last decade have increased the diversity and information density of these media, adding several intermediate layers to the storage hierarchy. This has been accompanied by a significant reduction in storage costs, as more bits are stored in the same volume. For example, solid-state hard drive storage costs have decreased at 37% CAGR from 2009 to 2019. These advances have enabled the rise of cloud-based architectures where storage and computation are done in remote data centers owned by third parties, thus lowering capital costs, simplifying scaling, and expanding data access.
However, industry forecasts and technical road maps suggest storage density is reaching a plateau as media approach physical scaling limits. In addition, supply chains for raw materials present challenges that limit capacity scaling, and pressures on margins can prohibit storage manufacturers from meeting demand. Forecasts suggest this will lead to an increase in storage costs per bit that may drastically raise the total cost of data ownership in the near future.
Developing An Effective Data Storage Strategy
Given anticipated TCO increases, data prioritization is an essential first step to managing data storage. This typically begins with a view from the future, where business use cases, business goals (e.g., costs, revenue, and value), and subject matter knowledge are used to identify products and services. Next is identifying data assets necessary to enable these products and services and the capabilities (e.g., scalability and efficiency, security and controls, and reliability) required to identify and manage these assets. This process of working backward concludes with strategic choices in foundational services and data architectures, including the storage platform (i.e., on-premises vs. cloud vs. hybrid), analytic engines, and the storage technology blend at each tier.
Organizations typically store data in multiple formats and across sources with various storage technologies, leading to a complex landscape with limited understanding of trusted data. Drawing value from these architectures may require data replication across the organization and the need to maintain multiple (potentially overlapping) tools, resulting in increased costs, such as resource consumption for maintenance. These challenges can be overcome with an approach to connect this data and make them accessible from a common tool.
Data integration and virtualization can help provide a unified view of data, whether on-premises or in the cloud. These approaches enable computing and analytics across all storage environments with advanced analytic engines (e.g., machine learning, deep learning, and AI) and reduce the overhead associated with reporting, regulation, compliance, and security.
A key aspect of future-forward strategy development is identifying emerging technologies with the potential to help companies store ever-growing data sets and cut costs. Examples of such burgeoning technologies with ultra-high density and minimal at-rest costs include DNA data storage, through storing digital bits in synthetic molecules; five-dimensional optical storage of data in nanoscale materials; and holographic storage of data in rewritable crystals.
These technologies address challenges in both the cold/archive and warm storage tiers by taking advantage of nanoscale phenomena to increase data density and longevity beyond the physical limits of current semiconductor and magnetic media. These attributes have been demonstrated at the prototype level, and modeling indicates they can be competitive on TCO over relevant time scales. However, challenges remain in demonstrating the ability to scale and the capacity for incorporation into modern architectures. Regardless, a sound data strategy will track advancement in these and related technologies to help establish that implementations are “future proof.”
How To Find The Best Data Storage Strategy For Your Organization
Data storage approaches may need to be forward-looking, tailored your organization’s needs, and capable of enduring far into the future, as data storage lifetimes can range from decades up to a century. For example, in the U.K., the Health and Social Care Records Practice Code of Practice 2016 specifies that anyone working with or in the National Health Service (NHS) is required to keep medical records for 20 years after the last contact with a patient — eight years after death or up to 25 years after the birth of the last child. In this new data ecosystem, CEOs, CIOs, and IT leadership will require a thorough understanding of the technology landscape and a data-driven technical approach to identify, select, and implement the best strategy and solution for their organization.
In this landscape, executives may want to ask the following questions:
- What are the revenue, cost, and value goals for the organization?
- Where is the opportunity? Are there new use cases that can drive data monetization opportunities? Do these require new or modified capabilities?
- What strategic partnerships can be used to advance the technology?
- What risks are involved, and how can they be managed?
Once new storage architectures are in place, companies may need to monitor the impact on their organization to both determine whether the change successfully meets their goals and when to consider implementing next-generation storage systems. While the primary metric is TCO over the storage lifetime, business-related KPIs can include storage capacity and density, increased data monetization revenue, format migration cadence, and integration with existing storage architecture.
Companies often employ legacy systems to manage their data, and increasing costs tied to the emerging business landscape are leading to a storage capacity gap. Novel data strategy approaches can decrease costs, introduce new ways of generating revenue, and incorporate innovative technologies to provide future-proof solutions for organizations to address this imminent data storage need.
About The Author:
Thomas Armel, Ph.D., is a founding partner of the Quantitative Strategies and Solutions practice at EY-Parthenon. He has more than 20 years of consulting experience across biotechnologies, biomanufacturing, medical devices, diagnostics, vaccines, and therapeutics. He holds a Ph.D. in molecular, cellular, and developmental biology with a graduate certificate in biophysics from the University of Colorado at Boulder and a BA in chemistry-biology from Whitman College.
The views expressed by the authors are not necessarily those of Ernst & Young LLP or other members of the global EY organization.